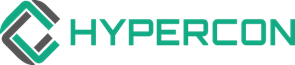
Initial situation
Hypercon Solutions UG is developing a plant concept for the automated production of UHCP building materials (ultra-high-performance concrete). In the individual automated plants, sensors collect and process data during the production process. The collected data from all plants will be used in the future to develop solutions with AI methods (artificial neural networks, ANN) that optimize the UHPC recipes used and/or the production process in terms of quality and cost-effectiveness, among other things.
Problem
At the time of conducting the QuickCheck, the systems are still under construction. Therefore, no existing information technology infrastructure can be taken into account. At the same time, there are no restrictions in this respect. Accordingly, no data sets are available for the application of concrete methods.
Therefore, this QuickCheck was focused to find an approach that would allow the IT infrastructure, as well as the AI component, to be developed in parallel with the construction of the system. Aspects such as scalability, sustainability and modularity of the solution were taken into consideration.
Solution
The proposed approach in this QuickCheck consisted of two detailed investigations into the key issues of designing an AI system for production optimization and a matching IT infrastructure.
QuickCheck results
For this use case, the scope of the QuickCheck was, to investigate possibilities for process optimization using AI. By decomposing the production process into single steps and identifying the parameters of each, it was possible to transform the posed problem into an AI problem. To address it, approaches for the construction of an AI-supported production optimization were developed. It was outlined how an AI system consisting of an optimizer and a prediction model can be built. Methods for the realization of the system components were proposed and explained. The generation of an initial database was identified as a particular challenge. As a possible solution to this challenge, it is explained how simulation tools can be used to gather training data for the prediction model. A systematic approach to building a data processing pipeline was proposed for connecting the AI system to real-world processes and for collecting operational data. The pipeline should evolve dynamically with the development progress of the system and be adaptable during operation.
In addition to the substantive results presented, suitable funding measures for further pursuit of the project were researched and presented.